Addressing Challenges In Automated Visual Inspection Of Lyophilized Vials
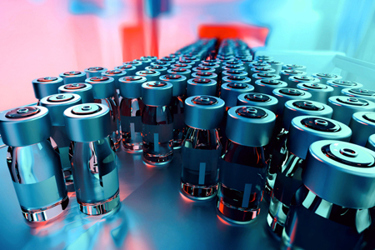
Table of Contents
Image Acquisition Challenges in Automated Visual Inspection
High-quality images are fundamental for successful automated visual inspection. Several factors can compromise image quality, leading to inaccurate defect detection.
Lighting and Shadow Effects
Variations in lighting are a major source of error in automated visual inspection of lyophilized vials. Shadows cast by the vial itself or surrounding equipment can obscure defects, leading to false negatives. Inconsistencies in lighting intensity can also affect the accuracy of color and contrast analysis.
- Need for consistent, optimized lighting: Implementing a carefully controlled lighting system is crucial. This might involve using multiple light sources to minimize shadowing.
- Importance of diffuse lighting techniques: Diffuse lighting helps to reduce harsh shadows and provides more even illumination across the vial's surface.
- Use of advanced imaging techniques like structured lighting: Structured lighting projects patterns onto the vial's surface, enabling three-dimensional analysis and improved defect detection. This technique can effectively reveal subtle surface imperfections often missed with traditional lighting. The use of polarization filters can further enhance image quality by reducing glare and reflections.
The selection of appropriate lighting is crucial for maximizing the contrast between the vial and potential defects, thereby improving the accuracy of the automated visual inspection process. Incorrect lighting setups can lead to both false positives (identifying defects where none exist) and false negatives (missing actual defects).
Variability in Vial Appearance
Lyophilized vials exhibit natural variability in appearance. Differences in fill levels, stopper position, and vial clarity introduce significant challenges for automated image analysis. These variations can be subtle, yet they can significantly impact the accuracy of the inspection system.
- Need for robust image pre-processing: Sophisticated image pre-processing techniques are essential to correct for these variations. This may involve algorithms that normalize fill levels, adjust for stopper variations, and compensate for inconsistencies in vial clarity.
- Importance of machine learning algorithms to handle variations: Machine learning algorithms, particularly deep learning models, can be trained on a large dataset of vial images representing the full range of normal variations. This allows the system to learn and adapt to subtle differences, improving its ability to discriminate between acceptable variations and actual defects.
- Calibration procedures to minimize variability: Regular calibration of the automated visual inspection system is crucial to ensure consistent performance. Calibration involves adjusting the system's parameters to account for changes in lighting, temperature, and other environmental factors that might impact image quality.
Addressing vial-to-vial variability requires a multi-faceted approach that integrates advanced image processing, machine learning, and rigorous calibration procedures.
Defect Detection and Classification Challenges
Accurately detecting and classifying defects in lyophilized vials is a significant challenge. Many defects are subtle and require advanced image analysis techniques to identify reliably.
Subtle Defect Identification
Many defects in lyophilized vials, such as cracks, particulate matter, or discolorations, are extremely subtle and difficult to detect with the naked eye. These defects, while seemingly minor, can compromise the quality and safety of the pharmaceutical product.
- Need for high-resolution imaging: High-resolution imaging systems are necessary to capture the fine details of these subtle defects.
- Application of advanced image processing algorithms: Advanced algorithms, such as edge detection, texture analysis, and pattern recognition, are crucial to identify subtle variations in image texture, color, and shape that might indicate a defect.
- Development of sophisticated machine learning models for defect classification: Machine learning models, trained on a large and diverse dataset of images, can significantly improve the accuracy of defect classification.
Effective identification of subtle defects requires a combination of high-resolution imaging capabilities, sophisticated image processing algorithms, and robust machine learning models trained to recognize a wide range of subtle defects.
False Positive and False Negative Minimization
Finding the right balance between sensitivity and specificity is critical. High sensitivity reduces the risk of missing defects (false negatives), while high specificity minimizes the rejection of good vials (false positives). Both scenarios can lead to considerable costs and delays.
- Use of multi-stage inspection processes: A multi-stage approach, where images are analyzed using multiple algorithms and criteria, can help to reduce both false positives and false negatives.
- Implementation of quality control measures: Regular quality control checks on the automated visual inspection system help to ensure accurate and consistent performance.
- Regular system calibration and maintenance: Preventative maintenance and regular recalibration ensure the system's continued accuracy and reliability, minimizing errors over time.
Minimizing errors requires careful design and implementation of the automated visual inspection system, integrating multiple detection methods, and incorporating robust quality control procedures.
System Integration and Validation Challenges
Successfully implementing automated visual inspection requires seamless integration with existing production lines and strict adherence to regulatory requirements.
Integration with Existing Production Lines
Integrating automated visual inspection systems into existing pharmaceutical production lines can be complex and require careful planning. Issues such as system footprint, interface compatibility, and data integration need careful consideration.
- Considerations for system footprint: The size and physical layout of the automated visual inspection system must be compatible with the available space in the production line.
- Interface compatibility with existing systems: The system must be able to seamlessly integrate with existing equipment, such as conveyor systems and data management systems.
- Software compatibility and data integration: The software controlling the automated visual inspection system must be compatible with existing software and capable of integrating with existing data management systems.
Careful planning and execution are crucial for a smooth integration of the automated visual inspection system into the overall production process.
Validation and Regulatory Compliance
Meeting stringent regulatory requirements for pharmaceutical manufacturing is paramount. Validation of the automated visual inspection system is crucial for ensuring compliance and demonstrating the system's reliability.
- Importance of thorough validation protocols: A comprehensive validation plan is required to demonstrate that the system meets all regulatory requirements. This involves rigorous testing and documentation.
- Documentation requirements for regulatory bodies: Detailed documentation is required to satisfy regulatory agencies, such as the FDA. This includes design specifications, test results, and maintenance records.
- Maintaining comprehensive audit trails: A complete and auditable trail of all system operations is necessary to ensure traceability and compliance.
Ensuring regulatory compliance requires a thorough understanding of the relevant regulations and a commitment to rigorous validation and documentation practices.
Conclusion
Automated visual inspection of lyophilized vials offers significant advantages in terms of speed, accuracy, and consistency compared to manual inspection. However, addressing challenges related to image acquisition, defect detection, and system integration is crucial for successful implementation. By utilizing advanced imaging techniques, sophisticated algorithms, and rigorous validation procedures, the pharmaceutical industry can overcome these hurdles and leverage the benefits of automated visual inspection for improved product quality and enhanced production efficiency. Investing in robust and reliable automated visual inspection systems is essential for ensuring the quality and safety of lyophilized pharmaceuticals. Further research and development in this area are needed to continually improve the capabilities and reliability of automated visual inspection of lyophilized vials. Choosing the right system and implementing it effectively is crucial for maximizing the return on investment in automated visual inspection.
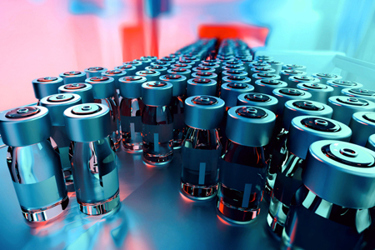
Featured Posts
-
The Challenge Season 41 Spoilers A Look At The Eliminations
May 11, 2025 -
Stadium Track Renovation In Time For Championships
May 11, 2025 -
Visita Familiar De Boris Johnson A Texas Se Ve Interrumpida Por Ataque De Avestruz
May 11, 2025 -
Ufc 315 Early Predictions Who Will Win Expert Analysis And Breakdown
May 11, 2025 -
The Enduring Appeal Of Meeting Shane Lowry
May 11, 2025
Latest Posts
-
Payton Pritchards Career Feat A Testament To His Roots
May 12, 2025 -
Payton Pritchard Analyzing His Breakout Year And Nba Sixth Man Of The Year Chances
May 12, 2025 -
The Impact Of Childhood On Payton Pritchards Basketball Achievements
May 12, 2025 -
The Making Of A Sixth Man Payton Pritchards Path To A Breakout Nba Season
May 12, 2025 -
Celtics Division Championship Secured By Dominant Performance
May 12, 2025