AI And Wildlife Conservation: Progress And Perils
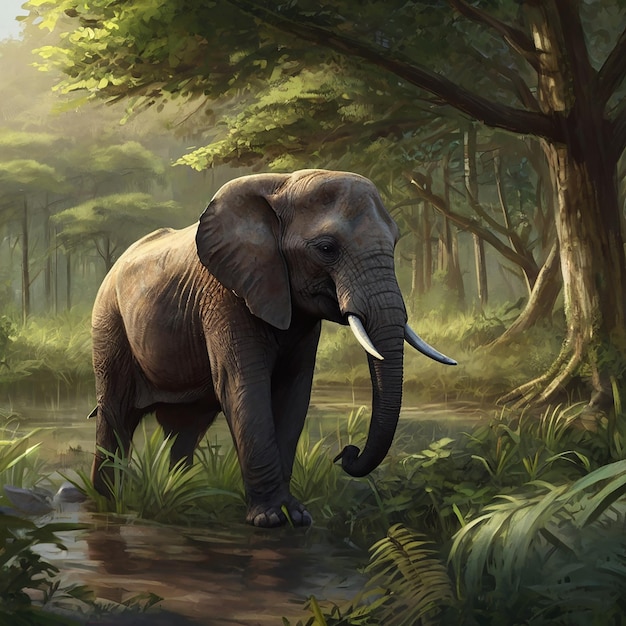
Table of Contents
Wildlife populations are plummeting at an alarming rate. The World Wildlife Fund reports a 68% average decline in monitored wildlife populations between 1970 and 2016 – a stark testament to the devastating impact of human activities. This crisis demands innovative solutions, and increasingly, AI and wildlife conservation are becoming inextricably linked. This article explores the burgeoning role of artificial intelligence in addressing critical conservation challenges, examining both the remarkable progress and the potential pitfalls inherent in this rapidly evolving field. We will delve into the promising applications of AI, while also critically analyzing the ethical and practical considerations that must guide its development and deployment.
H2: AI-Powered Solutions for Wildlife Monitoring and Protection
AI is revolutionizing how we monitor and protect wildlife. Sophisticated technologies are offering unprecedented insights into animal behavior, habitat degradation, and poaching activities.
H3: Real-time Tracking and Surveillance
The integration of AI with advanced tracking technologies is transforming wildlife monitoring. Drones equipped with AI-powered image recognition can survey vast areas, identifying individual animals, assessing population sizes, and monitoring habitat health far more efficiently than traditional methods. Similarly, camera traps, strategically placed in key locations, capture images and videos that AI algorithms can analyze to identify specific species, track individual animals, and detect poaching activity in real-time. Sensor networks, deployed across protected areas, gather environmental data – temperature, humidity, and movement – that AI can analyze to predict animal behavior and identify potential threats.
- Examples of successful AI applications:
- The use of AI to identify individual rhinos from their unique horn shapes, enabling precise monitoring of their movements and protection from poaching.
- Predictive modeling based on historical poaching data and environmental factors, allowing rangers to proactively deploy resources to high-risk areas.
- Real-time alerts triggered by AI-powered systems, notifying rangers of suspicious activity, such as the presence of vehicles in restricted zones.
H3: Analyzing Large Datasets for Conservation Insights
AI's ability to process massive datasets is invaluable for conservation. Satellite imagery, environmental sensor data, citizen science observations, and genetic data provide a wealth of information. AI algorithms can analyze this data to uncover complex patterns, predict threats, and optimize conservation strategies. This "AI-driven conservation analytics" allows for more informed decision-making, ultimately leading to more effective conservation outcomes.
- Examples of AI applications:
- Habitat analysis using satellite imagery to identify areas of suitable habitat for endangered species and monitor habitat fragmentation.
- Species distribution modeling to predict the future distribution of species based on climate change projections and other environmental factors.
- Predictive modeling to anticipate disease outbreaks based on environmental factors and animal movement patterns.
H2: Challenges and Ethical Considerations in AI for Wildlife Conservation
While AI offers immense potential, its application in wildlife conservation is not without challenges and ethical considerations.
H3: Data Bias and Algorithmic Fairness
The accuracy and effectiveness of AI depend heavily on the quality and representativeness of training data. "AI bias in conservation" can arise from an overrepresentation of certain species, geographical limitations in data collection, or biases in the selection of features used to train algorithms. This can lead to skewed results and potentially harmful conservation decisions. Algorithmic fairness requires careful consideration of data sources and rigorous validation of AI models to mitigate bias.
- Examples of potential biases:
- An AI model trained primarily on data from well-studied species might be less accurate in identifying less-studied or rarer species.
- A model trained on data from a specific region might not generalize well to other regions with different ecological conditions.
H3: Privacy Concerns and Data Security
The collection and use of data on wildlife often involves sensitive information about both animals and potentially humans. "Data security in wildlife conservation" is paramount. Robust data privacy regulations and secure data storage protocols are crucial to prevent unauthorized access and misuse of sensitive information. Responsible data handling practices must be implemented to ensure ethical and legal compliance.
- Key considerations:
- Compliance with data privacy regulations (e.g., GDPR).
- Secure data storage and transmission using encryption techniques.
- Transparent data governance policies.
H3: Technological Limitations and Accessibility
Current AI technology has limitations. High computational costs, the need for significant computing power, and a lack of robust algorithms for certain tasks hinder the widespread adoption of AI in resource-constrained settings. "AI accessibility in conservation" is a major challenge, requiring efforts to develop more efficient and affordable AI tools suitable for use in diverse environments.
- Examples of limitations:
- The high cost of training and deploying complex AI models can be prohibitive for organizations with limited budgets.
- The need for specialized expertise to develop, deploy, and maintain AI systems can be a barrier to adoption.
Conclusion:
AI presents both remarkable opportunities and significant challenges for wildlife conservation. AI-powered wildlife monitoring tools offer unprecedented capabilities for tracking animals, detecting poaching, and analyzing vast datasets to gain valuable insights. However, careful consideration of data bias, privacy concerns, and technological limitations is essential to ensure the responsible and ethical application of AI in this critical field. The future of AI-driven wildlife conservation hinges on fostering responsible innovation, developing accessible and robust AI tools, and promoting ethical data practices. We must all work together to support organizations developing ethical AI tools and advocate for responsible innovation in this vital area. Let us embrace the power of AI and wildlife conservation to safeguard our planet's biodiversity for generations to come.
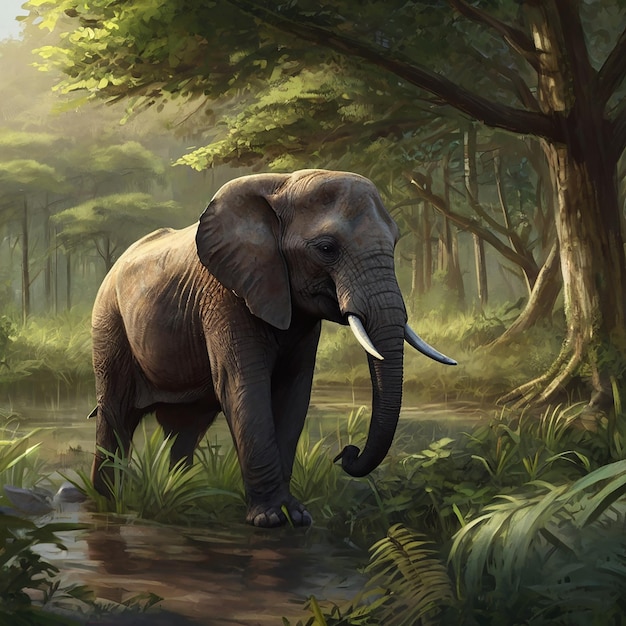
Featured Posts
-
Cole Ragans And The Royals Dominant Bullpen Performance Against The Brewers
Apr 23, 2025 -
Brewers Historic Nine Stolen Bases Power Blowout Win Over As
Apr 23, 2025 -
Cybercriminal Nets Millions Through Executive Office365 Compromises
Apr 23, 2025 -
Bof A Says Dont Worry About Stretched Stock Market Valuations
Apr 23, 2025 -
M3 As Autopalya Hetekig Tarto Forgalomkorlatozasok
Apr 23, 2025