AI Digest: Transforming Scatological Data Into Engaging Podcast Content
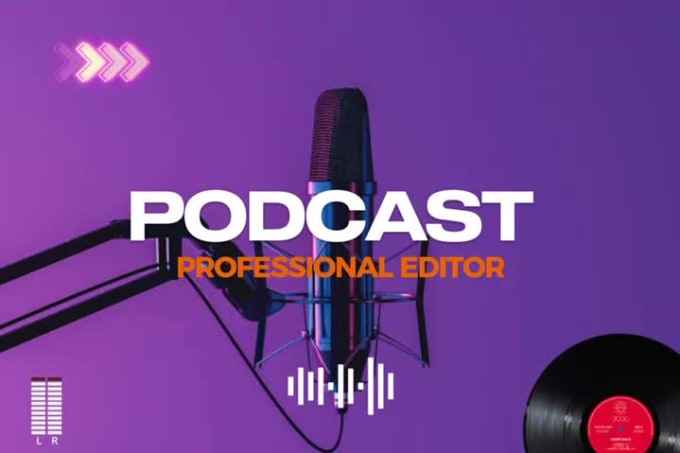
Table of Contents
Data Acquisition and Cleaning
The journey of transforming scatological data into compelling podcast content begins with acquiring and meticulously cleaning the raw data. This crucial first step ensures the accuracy and reliability of subsequent AI analysis.
Sourcing Scatological Data
Scatological data, while unconventional, comes from diverse sources. Identifying and accessing these sources is the first hurdle.
- Wastewater Treatment Plants: These plants are goldmines of scatological data, providing real-time information on the composition of wastewater. Access often requires navigating complex regulations and obtaining necessary permissions.
- Epidemiological Studies: Public health organizations and research institutions conduct epidemiological studies that often include scatological data. These studies provide valuable insights into disease outbreaks and public health trends. However, accessing this data might involve navigating IRB approvals and data sharing agreements.
- Social Media Sentiment Analysis: While seemingly unrelated, social media sentiment analysis can indirectly provide valuable contextual data. Analyzing public discourse around sanitation, hygiene, or related health issues can offer supplementary insights to the raw scatological data. This requires sophisticated natural language processing (NLP) techniques.
- Challenges: Data acquisition often faces significant hurdles. These include navigating complex legal frameworks related to data privacy (like HIPAA), obtaining informed consent where relevant, and dealing with inconsistencies in data formats across different sources.
Data Preprocessing and Cleaning
Raw scatological data is rarely ready for AI analysis. Significant preprocessing and cleaning are essential to ensure data quality.
- Handling Missing Data: Missing data points are common. Strategies like imputation (filling in missing values based on statistical methods) or removal of incomplete records are employed depending on the extent and nature of missing data.
- Outlier Detection and Treatment: Outliers—extreme data points—can skew results. Methods like Z-score analysis or box plots are used to identify and either remove or transform outliers.
- Data Consistency: Ensuring data consistency across various sources is crucial. This involves standardizing units, formats, and codes to facilitate seamless analysis.
- Tools and Techniques: Software like Python with libraries such as Pandas and Scikit-learn are commonly used for data cleaning and preprocessing.
AI-Powered Analysis and Interpretation
Once cleaned, the data is ready for AI-powered analysis. This involves employing sophisticated machine learning algorithms to uncover hidden trends and patterns.
Machine Learning Techniques
Various machine learning algorithms can effectively analyze scatological data.
- Anomaly Detection: Algorithms like Isolation Forest or One-Class SVM are useful in identifying unusual patterns indicative of potential health risks or environmental changes reflected in the wastewater.
- Predictive Modeling: Models like LSTM (Long Short-Term Memory) networks or RNNs (Recurrent Neural Networks) can be used to predict future trends based on historical scatological data, aiding in proactive public health interventions.
- Clustering Algorithms: Techniques like K-means or DBSCAN can group similar data points, identifying distinct patterns or subgroups within the dataset. This could reveal differences in water usage, diet, or disease prevalence within different populations.
Extracting Meaningful Insights
The real power of AI lies in its ability to extract meaningful insights from complex data.
- Disease Outbreak Detection: AI can identify early signs of disease outbreaks by detecting unusual changes in the composition of wastewater.
- Public Health Trends: Analyzing long-term trends can reveal valuable insights into the effectiveness of public health interventions or changes in lifestyle patterns affecting community health.
- Consumer Behavior Patterns: Indirectly, scatological data can provide insights into consumer behavior relating to diet and medication usage, providing a unique angle for podcast storytelling.
- Data Visualization: Tools like Tableau or Power BI are crucial for visualizing complex data into easily understandable charts and graphs, making it accessible to podcast listeners.
Creating Engaging Podcast Content
The final stage involves transforming the AI-driven insights into compelling podcast narratives.
Storytelling with Data
Data, however insightful, needs a compelling narrative to resonate with listeners.
- Narrative Strategies: Frame the data within a captivating narrative arc, highlighting the human element affected by the data insights.
- Audio Elements: Use sound effects, music, and interviews with experts to enhance the storytelling experience and provide additional context.
- Episode Structure: Organize each episode logically, building upon previous segments and providing a clear takeaway message.
Ethical Considerations and Responsible Data Handling
Ethical considerations are paramount when dealing with sensitive scatological data.
- Data Anonymization: Stringent anonymization techniques are essential to protect individual privacy.
- Informed Consent: Obtaining informed consent where applicable ensures ethical data collection and responsible usage.
- Data Privacy Regulations: Adherence to regulations like GDPR and HIPAA is crucial.
- Avoiding Bias: Careful attention must be paid to avoid bias in data interpretation and presentation.
Conclusion
AI is transforming the podcasting landscape by enabling the analysis of even unconventional datasets like scatological data. By leveraging the power of AI, we can unearth valuable insights from this often-overlooked resource, creating engaging and informative podcasts that address vital issues in public health, environmental science, and beyond. The process requires careful consideration of data acquisition, cleaning, ethical implications, and narrative construction. By embracing responsible AI practices, we can unlock the potential of scatological data analysis to tell compelling and impactful stories through podcasting. Start exploring the potential of AI and scatological data analysis for your next podcast project – the possibilities are vast!
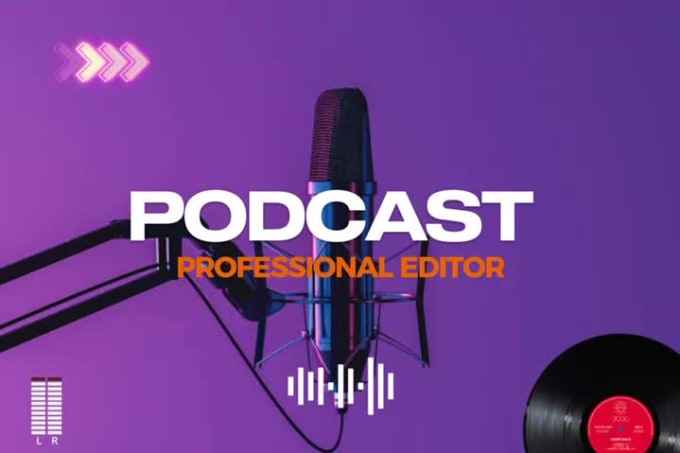
Featured Posts
-
Oh Jun Sung Triumphs In Chennai Wtt Star Contender
May 22, 2025 -
Jail Term For Tory Councillors Wife Over Hotel Fire Tweet Appeal Decision Expected
May 22, 2025 -
Taylor Swift And Blake Lively Exploring The Alleged Rift Caused By Legal Conflicts
May 22, 2025 -
Chinese Towers Partial Collapse Triggers Tourist Evacuation
May 22, 2025 -
Blake Livelys Alleged Blackmail Of Taylor Swift The Baldoni Conflict And Its Fallout
May 22, 2025
Latest Posts
-
Why Did Core Weave Inc Crwv Stock Jump Last Week
May 22, 2025 -
Tuesdays Core Weave Crwv Stock Dip Factors Contributing To The Decrease
May 22, 2025 -
Thursdays Core Weave Crwv Stock Decline A Detailed Explanation
May 22, 2025 -
Understanding Core Weaves Crwv Recent Stock Price Increase
May 22, 2025 -
Cong Dung Cua Hai Lo Vuong Tren Cong Usb Ban Can Biet Dieu Nay
May 22, 2025