AI's Cognitive Processes: A Closer Look At How Artificial Intelligence Works
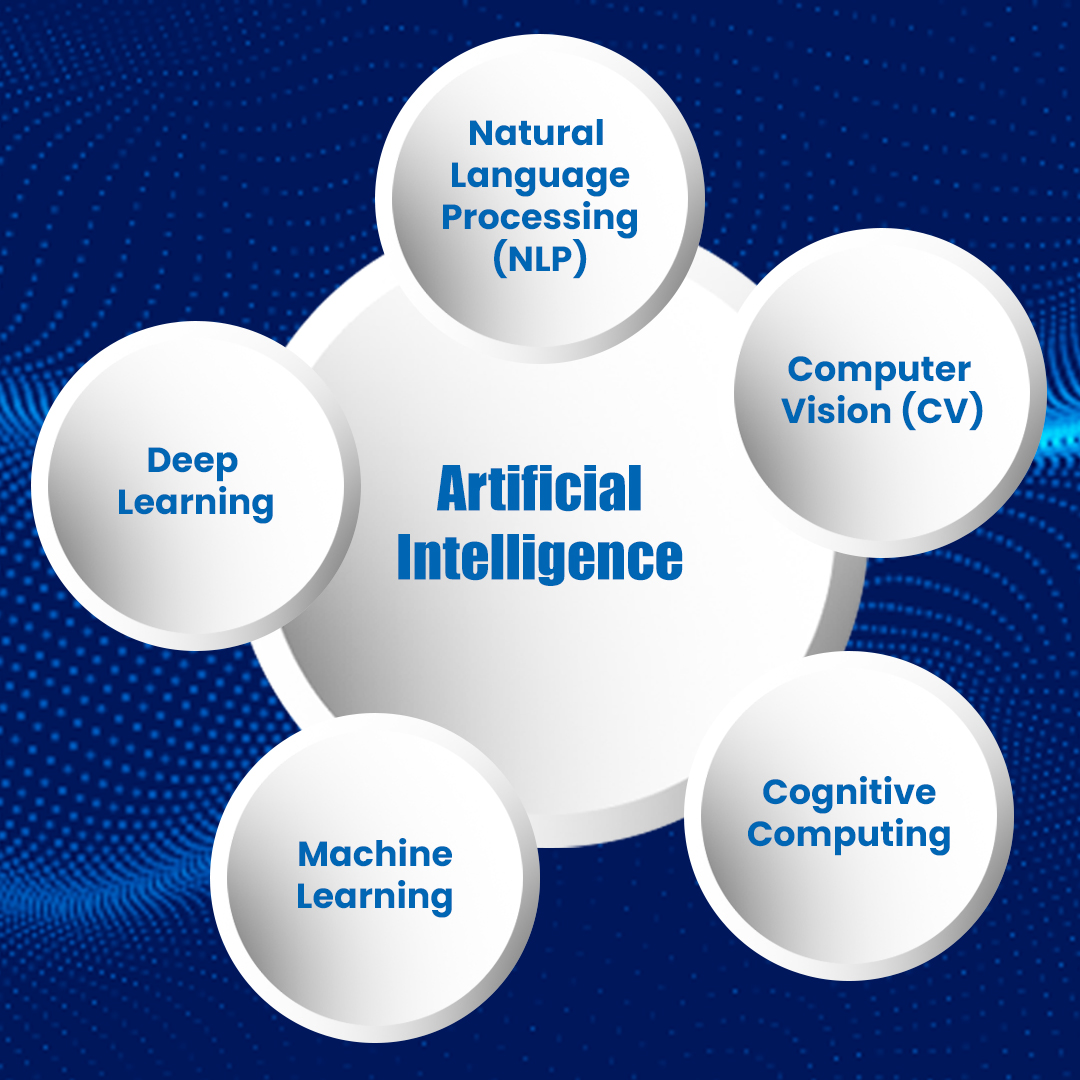
Table of Contents
Understanding Machine Learning as a Core Cognitive Process
Machine learning forms the bedrock of many AI systems, enabling them to learn from data without explicit programming. This learning process can be categorized into several key types:
Supervised Learning: Learning from Labeled Data
Supervised learning algorithms learn from labeled datasets, meaning each data point is tagged with the correct answer. The algorithm identifies patterns in the data to predict outcomes for new, unseen data.
- Example: Imagine training an AI to identify cats in images. You would feed the algorithm thousands of images, each labeled as either "cat" or "not cat." The algorithm learns to identify features associated with cats (e.g., pointy ears, whiskers, fur) and uses these features to classify new images.
- Keyword Integration: This process utilizes supervised learning algorithms, analyzing training data for classification and regression tasks. The accuracy depends heavily on the quality and quantity of the training data.
Unsupervised Learning: Finding Patterns in Unlabeled Data
Unlike supervised learning, unsupervised learning deals with unlabeled data. The algorithm's task is to identify inherent structures, patterns, and relationships within the data without any prior knowledge.
- Example: Consider customer segmentation in marketing. An unsupervised learning algorithm can analyze customer purchasing behavior, grouping similar customers together based on their spending habits, even without pre-defined customer categories. This helps tailor marketing strategies effectively.
- Keyword Integration: Common techniques include clustering algorithms to group similar data points, dimensionality reduction to simplify data, anomaly detection to identify outliers, and self-organization maps to visualize data structures.
Reinforcement Learning: Learning Through Trial and Error
Reinforcement learning involves an agent learning to interact with an environment by trial and error. The agent receives rewards for desirable actions and penalties for undesirable ones, learning an optimal strategy to maximize cumulative rewards.
- Example: Many successful game-playing AIs utilize reinforcement learning. An AI playing chess, for instance, learns through countless games, adjusting its strategy based on the outcomes (rewards or penalties).
- Keyword Integration: Key concepts include the reward function, which defines what constitutes a good or bad outcome, state-action pairs representing the agent's actions in different situations, and algorithms like Q-learning and policy gradients which determine the agent's learning process.
The Power of Deep Learning in Mimicking Human Cognition
Deep learning is a subset of machine learning that utilizes artificial neural networks with multiple layers (hence "deep") to extract higher-level features from raw data. This allows for more complex pattern recognition and problem-solving capabilities.
Neural Networks: The Building Blocks of Deep Learning
Artificial neural networks (ANNs), the fundamental building blocks of deep learning, are inspired by the structure and function of the human brain. They consist of interconnected nodes (neurons) organized in layers, processing information through weighted connections and activation functions. Deep neural networks (DNNs) have many layers, enabling them to learn complex representations from data.
- Keyword Integration: Understanding the architecture of ANNs and DNNs, including the different types of neurons, layers (input, hidden, output), and activation functions (ReLU, sigmoid, etc.) is crucial to grasping deep learning's capabilities.
Convolutional Neural Networks (CNNs): Masters of Image and Video Processing
Convolutional Neural Networks (CNNs) are a specialized type of neural network particularly adept at processing visual data. They excel at tasks such as image recognition, object detection, and video analysis.
- Keyword Integration: CNNs use convolutional layers to extract features from images, making them ideal for computer vision applications and feature extraction.
Recurrent Neural Networks (RNNs): Processing Sequential Data
Recurrent Neural Networks (RNNs) are designed to handle sequential data, such as text and time series. They have internal memory, allowing them to consider previous inputs when processing current ones.
- Keyword Integration: RNNs are crucial for natural language processing (NLP) tasks like machine translation, speech recognition, and sentiment analysis; also useful in time series analysis for forecasting and pattern recognition.
Natural Language Processing (NLP) and Understanding Human Communication
Natural Language Processing (NLP) focuses on enabling computers to understand, interpret, and generate human language. This field is critical for building AI systems that can communicate and interact with humans naturally.
Text Analysis: Unraveling the Meaning of Text
NLP techniques enable computers to analyze textual data, extracting valuable insights and information.
- Keyword Integration: This involves sentiment analysis to determine the emotional tone of text, topic modeling to identify recurring themes, text summarization to condense large amounts of text, and named entity recognition to identify key people, places, and organizations.
Speech Recognition: Converting Speech to Text
Speech recognition technology converts spoken language into written text, enabling hands-free interaction with computers and other devices.
- Keyword Integration: This process uses acoustic modeling to analyze the sound waves and language modeling to interpret the linguistic structure, resulting in accurate speech-to-text conversion and voice recognition capabilities.
Chatbots and Conversational AI: Building Human-like Interactions
Conversational AI, powered by NLP, aims to create AI systems capable of engaging in natural, human-like conversations. Chatbots are a prime example, providing automated customer service or companionship.
- Keyword Integration: Key aspects include dialogue management to maintain context throughout a conversation, natural language generation (NLG) to produce coherent and grammatically correct responses, intent recognition to understand the user's goal, and advancements in conversational AI.
Conclusion
AI's cognitive processes, encompassing machine learning, deep learning, and natural language processing, are complex and interwoven. Understanding these processes is not only essential for developers and researchers but also for the general public to grapple with the ethical implications and future potential of this technology. The ability of AI to learn from data, recognize patterns, and even understand human language is transforming industries and our daily lives. Continue your exploration of AI's cognitive processes and discover the fascinating possibilities this field holds!
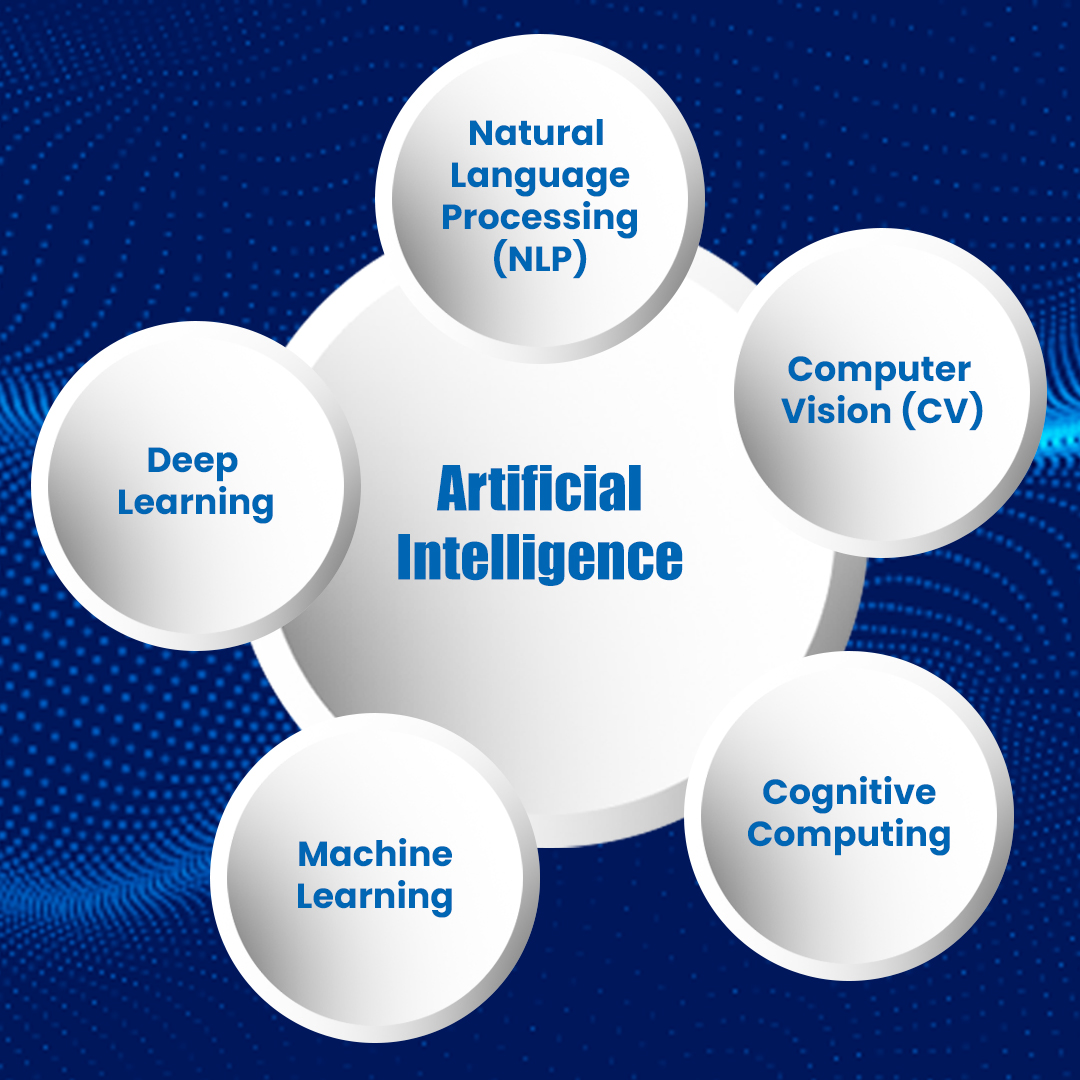
Featured Posts
-
Wrexham Afcs Rise Ryan Reynolds Cheers On The Teams Promotion
Apr 29, 2025 -
Arizona Speedboat Record Attempt Ends In Dramatic Flip
Apr 29, 2025 -
Boating Competition In Arizona Speedboats Record Attempt Ends In A Flip
Apr 29, 2025 -
Why Current Stock Market Valuations Shouldnt Deter Investors Bof A
Apr 29, 2025 -
Texas Woman Killed In Wrong Way Crash Near Minnesota North Dakota Border
Apr 29, 2025
Latest Posts
-
Ohio Train Derailment Investigation Into Long Term Toxic Chemical Presence In Buildings
Apr 29, 2025 -
Detailed One Plus 13 R Review A Budget Friendly Alternative To Pixel 9a
Apr 29, 2025 -
Choosing Between One Plus 13 R And Pixel 9a Performance Camera And Value
Apr 29, 2025 -
One Plus 13 R And Pixel 9a Feature By Feature Review And Recommendation
Apr 29, 2025 -
Is The One Plus 13 R Worth It Comparing It To The Google Pixel 9a
Apr 29, 2025