Enhancing The Reliability Of Automated Visual Inspection Systems For Lyophilized Vials
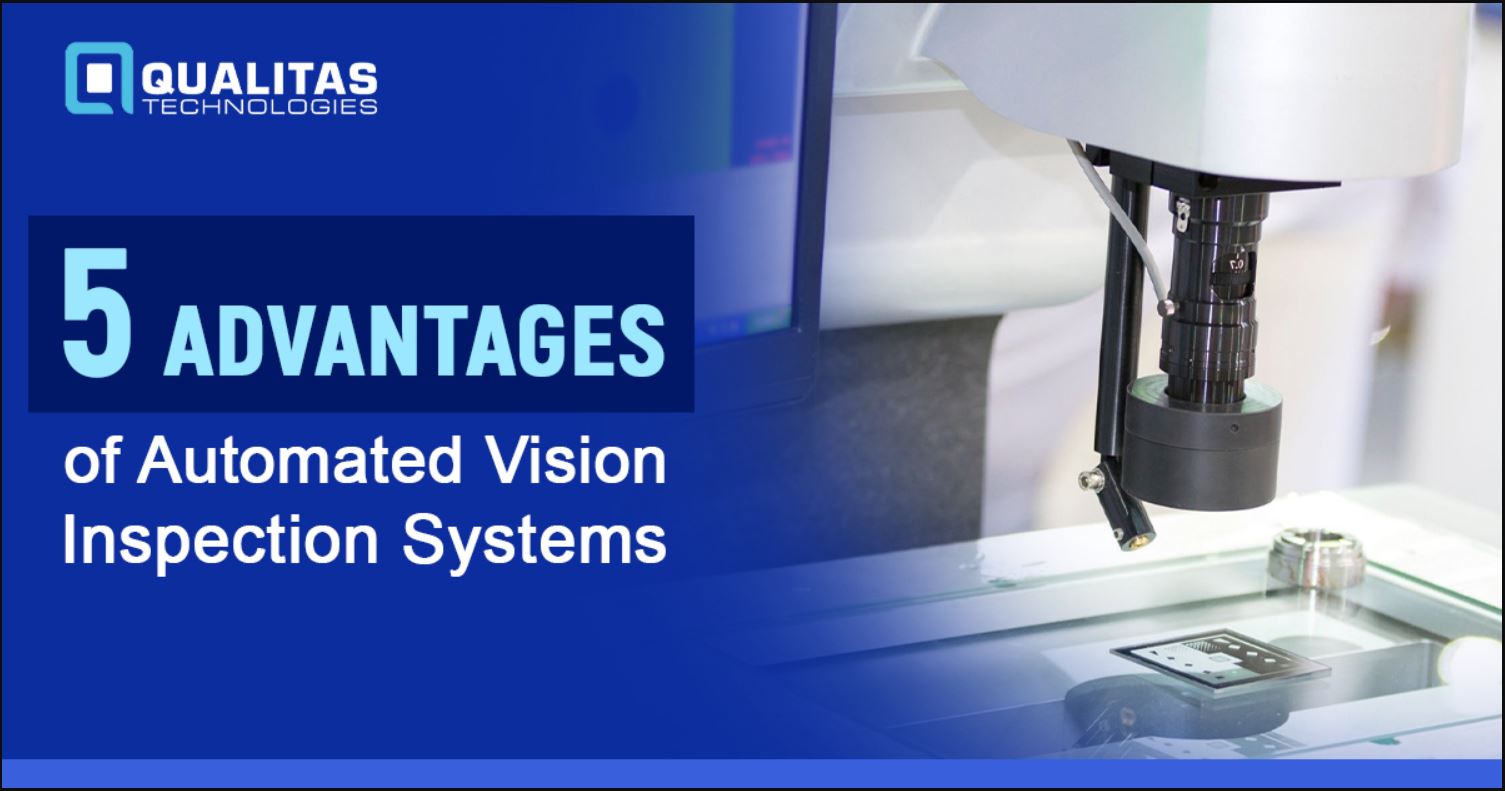
Table of Contents
Image Acquisition and Preprocessing Techniques for Enhanced Reliability
High-quality images are the foundation of any successful automated visual inspection system. Effective image acquisition and preprocessing are crucial for minimizing errors and maximizing the accuracy of defect detection in lyophilized vials.
Illumination Optimization
The choice of illumination significantly impacts image quality. Different lighting techniques offer distinct advantages and disadvantages:
-
Coaxial lighting: This method illuminates the vial from a direction directly opposite the camera. Coaxial lighting excels at highlighting surface imperfections, such as cracks, scratches, and particulate matter, by minimizing shadows and enhancing surface detail.
-
Diffuse lighting: Diffuse lighting uses a scattering technique to provide even illumination across the vial's surface. Diffuse lighting minimizes shadows, improving overall visibility, particularly for detecting defects within the lyophilized cake. However, it might reduce contrast for subtle surface flaws.
-
Ring lighting: Ring lights surround the vial, providing uniform illumination. Ring lighting provides even illumination, reducing artifacts and ensuring consistent image quality. However, it can be less effective in highlighting certain types of surface defects.
The optimal illumination strategy depends on the specific types of defects being targeted and the characteristics of the lyophilized vial. Careful experimentation and optimization are essential for achieving the best results.
Advanced Image Filtering and Enhancement
Raw images from automated visual inspection often contain noise and artifacts that can interfere with defect detection. Advanced image processing techniques are vital for enhancing image quality and improving the accuracy of defect identification. These techniques include:
-
Noise reduction algorithms: Algorithms like wavelet transforms and median filtering effectively minimize false positives caused by background noise. This improves the signal-to-noise ratio, allowing for more reliable defect detection.
-
Contrast enhancement: Techniques such as histogram equalization or adaptive contrast enhancement improve the visibility of subtle defects, making them easier to identify by the automated system.
-
Edge detection algorithms: Algorithms like Canny edge detection precisely locate the boundaries of defects, providing valuable information for accurate classification and measurement.
Defect Detection and Classification Algorithms
The effectiveness of an automated visual inspection system hinges on the accuracy and reliability of its defect detection and classification algorithms.
Machine Learning for Improved Accuracy
Machine learning algorithms, particularly deep learning models, are revolutionizing automated visual inspection.
-
Convolutional Neural Networks (CNNs): CNNs are particularly effective in identifying complex patterns and textures, making them ideal for detecting subtle defects in lyophilized vials that may be difficult to identify using traditional methods.
-
Support Vector Machines (SVMs): SVMs are efficient algorithms for classifying known defect types. SVMs provide efficient classification of known defect types, especially when dealing with large datasets.
-
Training Data: The performance of these machine learning models is directly dependent on the size and quality of the training data. Large, high-quality training datasets are crucial for optimal performance. The dataset needs to be representative of the various defects and variations that can occur in lyophilized vials.
Algorithm Optimization and Validation
Regular algorithm optimization and validation are crucial for maintaining the accuracy and reliability of the automated visual inspection system.
-
Algorithm Updates: Regular algorithm updates and retraining are vital to maintain accuracy as new defect types emerge or vial characteristics change.
-
Independent Dataset Validation: Performance should be validated using independent datasets and expert review. This ensures that the algorithm is generalizing well and not overfitting to the training data.
-
Statistical Process Control (SPC): Statistical process control (SPC) techniques can track algorithm performance over time, allowing for proactive identification and correction of any performance degradation. Metrics such as precision, recall, and F1-score are used to assess the algorithm’s performance.
Hardware Considerations for Robust Automated Visual Inspection
The choice of hardware significantly impacts the reliability and efficiency of the automated visual inspection system.
High-Resolution Imaging Systems
High-resolution imaging is critical for capturing fine details in lyophilized vials.
-
High Resolution: High resolution ensures the detection of even small defects, such as hairline cracks or microscopic particulate matter, which could impact product quality.
-
Appropriate Lenses: Appropriate lens selection minimizes distortion and maximizes image clarity, ensuring accurate defect detection and measurement.
Robotic Handling and Automation
Robotic systems play a crucial role in automating the vial handling process, improving throughput and minimizing human intervention.
-
Consistent Handling: Robotic systems ensure consistent and reliable vial handling, reducing variability and improving the overall reliability of the inspection process.
-
Reduced Human Error: Automation reduces the risk of human error and damage to vials, leading to increased efficiency and improved product quality.
Conclusion
Improving the reliability of Automated Visual Inspection Systems for Lyophilized Vials requires a holistic approach encompassing advanced image acquisition and preprocessing techniques, sophisticated defect detection algorithms, and robust hardware. By optimizing illumination, employing advanced image filtering and enhancement techniques, utilizing machine learning for accurate defect classification, and employing high-resolution imaging systems with robotic automation, manufacturers can significantly enhance the accuracy and efficiency of their quality control processes. The key takeaways are reduced costs, enhanced product quality, and increased patient safety. Implementing these advanced techniques in your Automated Visual Inspection Systems for Lyophilized Vials will significantly improve your quality control processes and contribute to the production of safe and effective lyophilized pharmaceuticals.
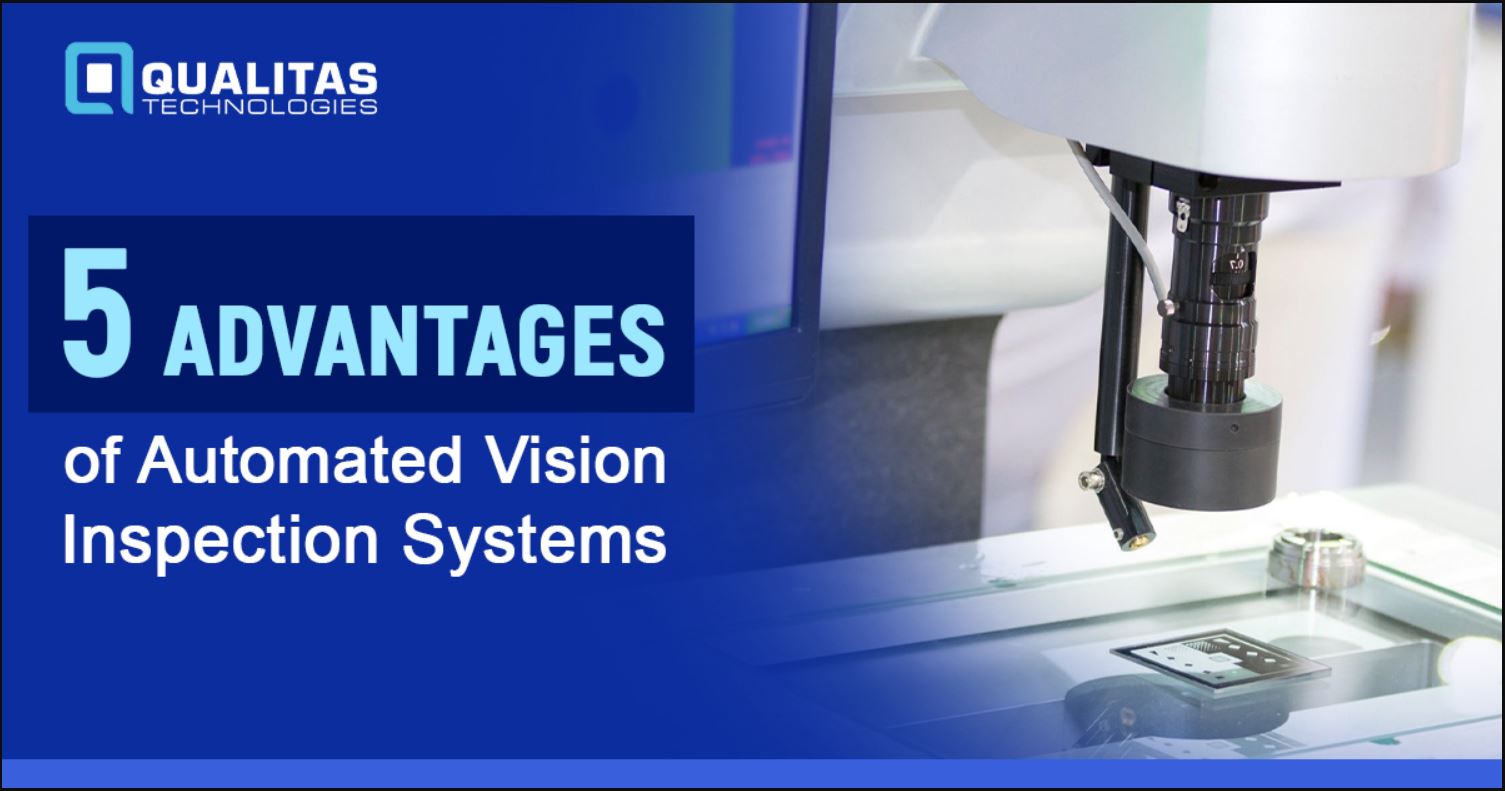
Featured Posts
-
Zabavniot Sostanok Na Nikola Okikj I Kevin Khart
May 11, 2025 -
Alex Winters Early Work Unveiling His Pre Freaked Mtv Sketches
May 11, 2025 -
Payton Pritchards Case For Sixth Man Of The Year
May 11, 2025 -
The Crime Thriller Sequel Stallone Almost Ruined
May 11, 2025 -
Jessica Simpson Reflects Career Insecurities And The Shadow Of Britney And Christina
May 11, 2025
Latest Posts
-
Us Ambassadors Statement On Canada Tariffs Partial Removal Possible
May 12, 2025 -
The Trump Tariff Impact Toyotas Heavy Burden Compared To Other Automakers
May 12, 2025 -
Canada Tariffs Us Ambassador Casts Doubt On Complete Removal
May 12, 2025 -
Analysis Toyotas Substantial Losses Due To Trump Era Tariffs
May 12, 2025 -
Trump Tariffs How Toyota Faced The Most Significant Losses
May 12, 2025