How AI Thinks: A Look At The Limitations Of Current Artificial Intelligence
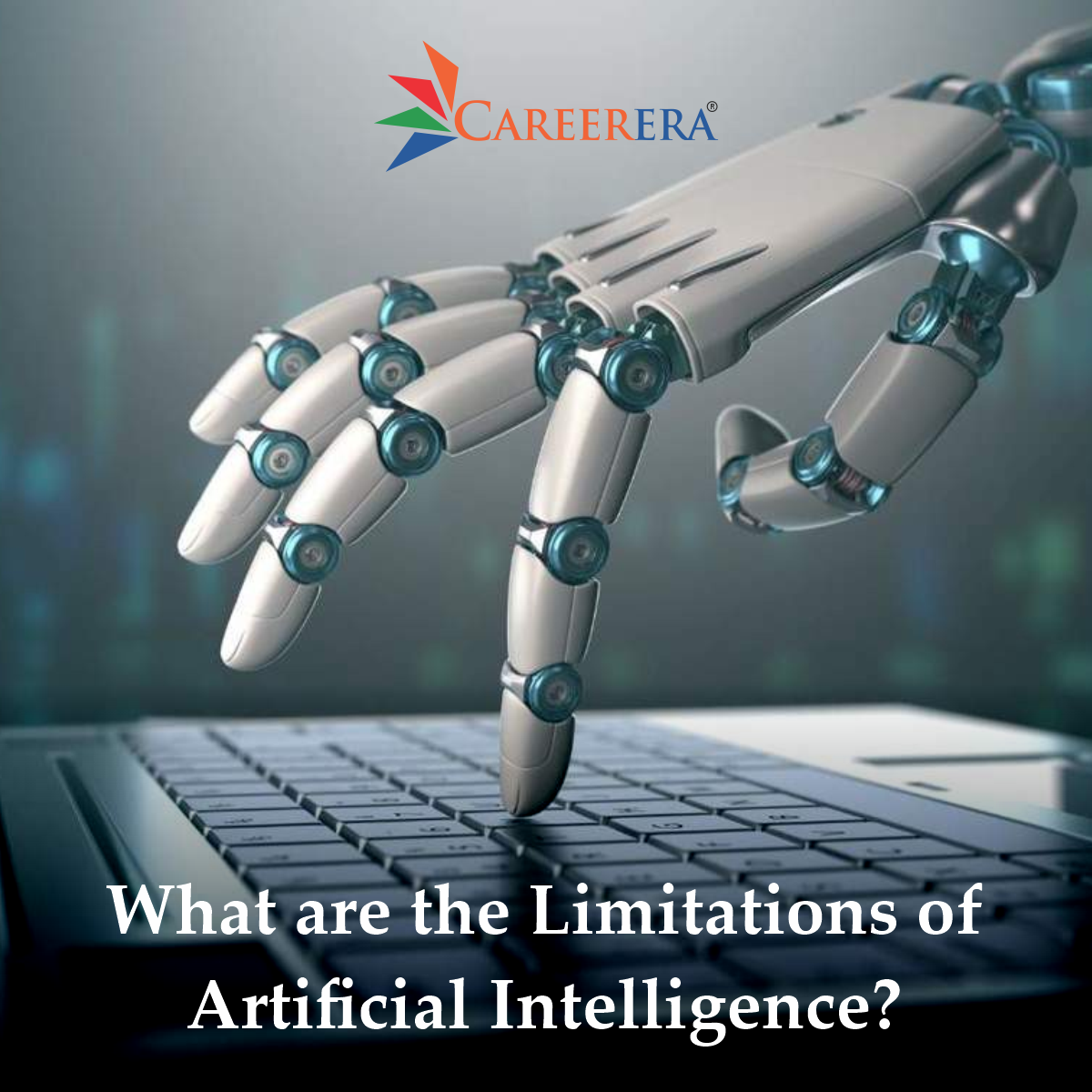
Table of Contents
Lack of Common Sense and Real-World Understanding
One of the most significant limitations of artificial intelligence is its persistent lack of common sense and real-world understanding. AI systems, even the most advanced, struggle with the nuanced context and implicit knowledge that humans effortlessly grasp.
The Problem of Context
AI often fails to understand context, leading to errors in various applications. This lack of contextual awareness is a major hurdle in achieving truly intelligent systems.
- Image recognition errors: An AI might misidentify a cat sitting on a laptop as a dog due to a lack of understanding of the surrounding objects and their relationships.
- Misinterpretations in natural language processing: An AI chatbot might misunderstand a sarcastic comment, responding literally instead of recognizing the intended meaning.
- Symbolic reasoning limitations: Humans excel at symbolic reasoning – using abstract symbols to represent and manipulate information. Most current AI systems lack this capability, restricting their ability to solve complex problems requiring abstract thought.
- Common sense knowledge representation: Representing common sense knowledge – the vast, implicit knowledge humans possess about the world – in a machine-readable format remains a significant challenge.
Limited Generalization Capabilities
Another key aspect of the limitations of artificial intelligence is its struggle with generalization. AI systems are often trained on specific datasets and struggle to apply their knowledge to slightly different scenarios or domains.
- Overfitting: An AI model might perform exceptionally well on the data it's trained on but fail miserably when presented with new, unseen data.
- Narrow vs. General AI: Current AI systems are largely "narrow" AI – designed for specific tasks. General AI, capable of performing a wide range of tasks, remains a distant goal.
- Transfer learning: Research into transfer learning – applying knowledge gained from one domain to another – offers potential solutions to this limitation, allowing AI to generalize more effectively.
The Data Dependency Problem
AI's reliance on vast amounts of data is a double-edged sword. While data fuels AI's capabilities, its limitations are directly tied to the quality and quantity of data available.
Bias in Training Data
Biases present in training data inevitably lead to biased AI outputs, perpetuating and even amplifying existing societal inequalities.
- Facial recognition bias: AI facial recognition systems have demonstrated bias against certain ethnic groups, highlighting the dangers of biased datasets.
- Loan application bias: AI algorithms used in loan applications have been shown to discriminate against certain demographic groups due to biases embedded in the training data.
- Recruitment process bias: AI-powered recruitment tools can perpetuate existing biases in hiring practices, leading to unfair and discriminatory outcomes.
- Diverse datasets: Creating diverse and representative datasets is crucial for mitigating bias and ensuring fairness in AI systems.
- Bias mitigation techniques: Researchers are developing techniques to identify and mitigate bias in AI algorithms, such as data augmentation and adversarial training.
The Need for Massive Datasets
Training effective AI models requires immense amounts of data, placing significant demands on computational resources and energy consumption.
- Computational resources: Training large AI models requires powerful computers and significant energy consumption.
- Data acquisition and labeling: Gathering, cleaning, and labeling large datasets is a time-consuming and expensive process.
- Data augmentation: Techniques such as data augmentation can help alleviate the need for massive datasets by artificially expanding the training data.
- Transfer learning: Transfer learning can reduce data requirements by leveraging knowledge learned from other tasks or domains.
Explainability and Transparency
A significant challenge in understanding the limitations of artificial intelligence is the "black box" problem – the difficulty in understanding the decision-making processes of complex AI models.
The "Black Box" Problem
Many AI systems operate as black boxes, making it difficult to understand why they arrive at particular decisions.
- Lack of explainability: AI systems making critical decisions, such as medical diagnoses or loan approvals, need to be explainable to ensure accountability and trustworthiness.
- Transparency in AI: Transparency is crucial for building trust and ensuring responsible use of AI systems.
- Explainability techniques: Techniques like LIME (Local Interpretable Model-agnostic Explanations) and SHAP (SHapley Additive exPlanations) are being developed to improve the explainability of AI models.
Ethical Considerations
The lack of transparency in AI systems raises significant ethical concerns, particularly regarding potential misuse and lack of control.
- AI in surveillance: The use of AI in surveillance raises concerns about privacy and potential for abuse.
- Autonomous weapons: The development of autonomous weapons systems presents profound ethical challenges, raising questions about accountability and the potential for unintended consequences.
- AI in decision-making: Using AI in high-stakes decision-making scenarios requires careful consideration of ethical implications and potential for bias.
- Ethical guidelines and regulations: The development and deployment of AI require robust ethical guidelines and regulations to mitigate potential risks.
Conclusion
In summary, the limitations of artificial intelligence are significant and multifaceted. The lack of common sense, the heavy reliance on data, and the opacity of many AI systems present considerable challenges. Understanding these limitations of artificial intelligence is crucial to avoid unrealistic expectations and to promote the responsible development of this transformative technology. By acknowledging these limitations, we can work towards building more robust, ethical, and beneficial AI systems for the future. Continue exploring the limitations of artificial intelligence to contribute to responsible innovation in this rapidly evolving field.
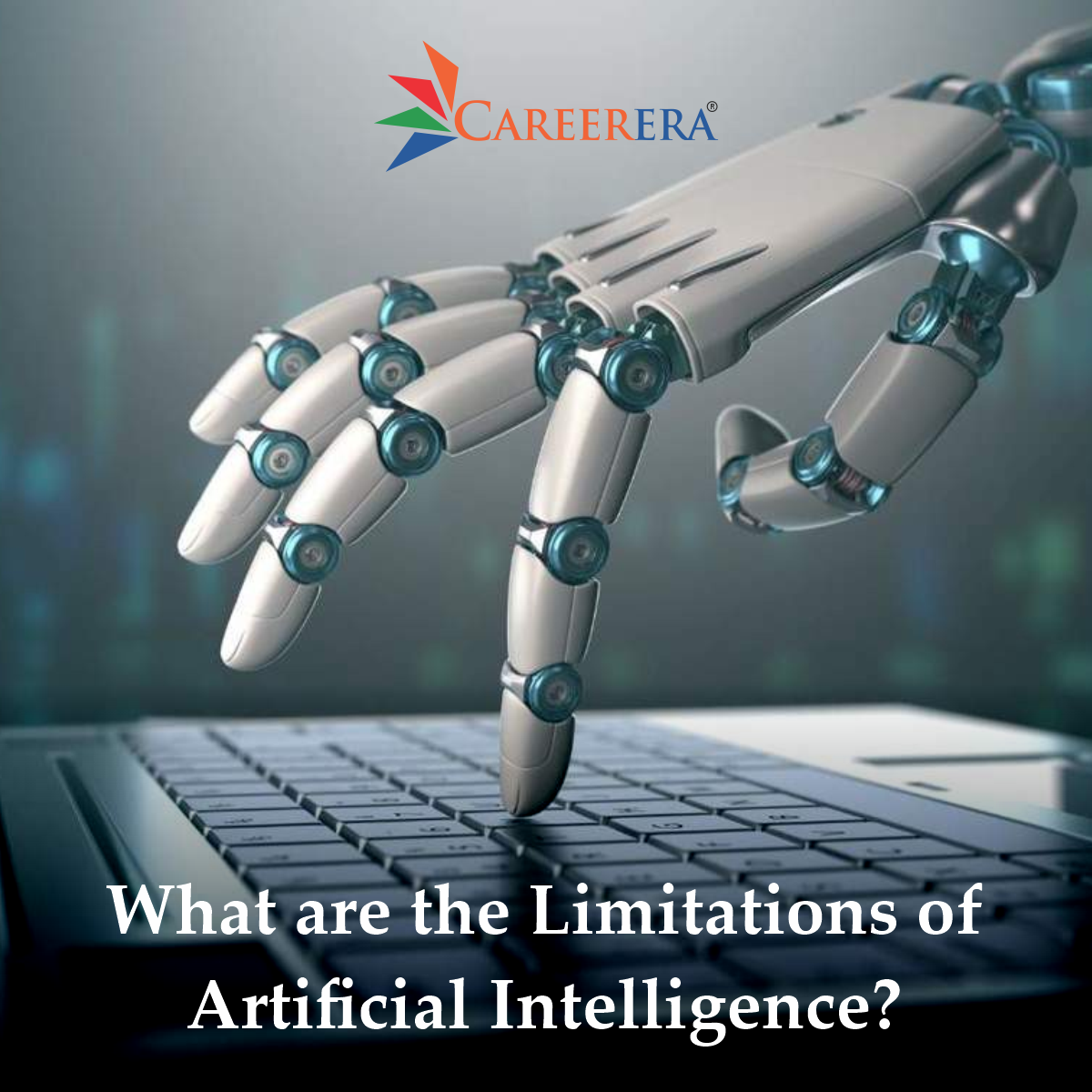
Featured Posts
-
European Power Prices Plunge Solar Surge Sends Prices Below Zero
Apr 29, 2025 -
Price Gouging Allegations Surface In La Following Devastating Fires
Apr 29, 2025 -
Mets Fall To Twins 3 6 In Series Showdown
Apr 29, 2025 -
The Countrys Evolving Business Landscape A Location Based Guide
Apr 29, 2025 -
Silver Lake Investment Fuels Khaznas Saudi Data Center Growth
Apr 29, 2025
Latest Posts
-
Choosing Between One Plus 13 R And Pixel 9a Performance Camera And Value
Apr 29, 2025 -
One Plus 13 R And Pixel 9a Feature By Feature Review And Recommendation
Apr 29, 2025 -
Is The One Plus 13 R Worth It Comparing It To The Google Pixel 9a
Apr 29, 2025 -
One Plus 13 R Review A Practical Assessment Against The Pixel 9a
Apr 29, 2025 -
One Plus 13 R Vs Pixel 9a A Detailed Comparison Review
Apr 29, 2025