How Outdated Business Apps Hamper AI Implementation
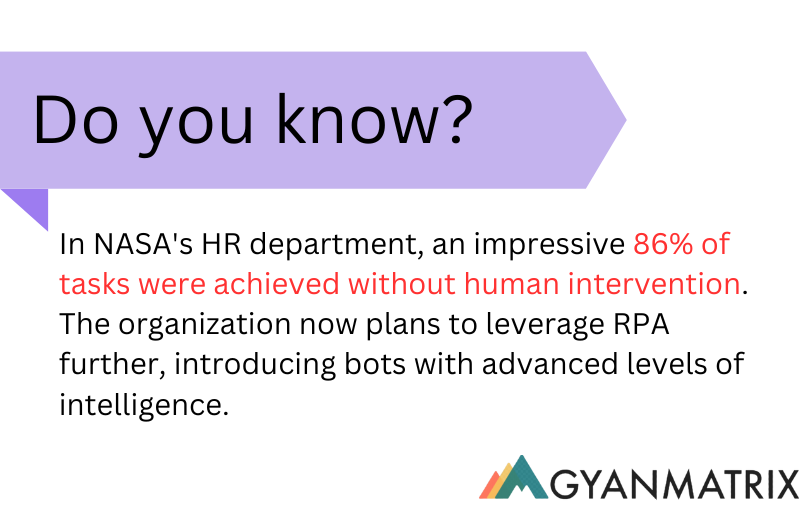
Table of Contents
Data Incompatibility and Integration Challenges
The foundation of any successful AI initiative is robust, reliable data. However, many organizations struggle with data incompatibility stemming from their reliance on outdated business applications. This leads to significant integration challenges, hindering the development and deployment of effective AI models.
Data Silos and Lack of Interoperability
Outdated systems often create data silos, where data is trapped in isolated compartments, making it incredibly difficult to gather the comprehensive datasets necessary for training and running AI algorithms. This fragmented data landscape presents numerous obstacles:
- Incompatible data formats: Different systems may use various formats (CSV, XML, proprietary formats), making it challenging to consolidate and analyze data effectively.
- Lack of APIs: The absence of Application Programming Interfaces (APIs) prevents seamless data exchange between systems, requiring manual intervention and increasing the risk of errors.
- Difficulties in data cleaning and transformation: Data from legacy systems is often dirty, inconsistent, and incomplete, necessitating extensive cleaning and transformation before it can be used for AI. This is a time-consuming and resource-intensive process.
Effective data integration strategies, including implementing robust ETL (Extract, Transform, Load) processes and data standardization techniques, are crucial for overcoming these challenges. Investing in modern data management platforms and APIs is key to breaking down data silos and unlocking the value of your data for AI.
Difficulty in Data Extraction and Processing
Extracting usable data from outdated systems is often a laborious and error-prone process. Many legacy applications lack the capabilities for automated data extraction, relying instead on manual methods:
- Manual data entry: This is time-consuming, prone to human error, and significantly slows down the AI development process.
- Inefficient reporting systems: Outdated systems may generate reports in incompatible formats or lack the flexibility to extract the specific data needed for AI.
- Lack of automation capabilities: The inability to automate data extraction and preprocessing severely impacts efficiency and scalability.
Implementing modern data extraction, cleansing, and preprocessing techniques, leveraging tools designed for data mining and ETL processes, can significantly improve the efficiency and accuracy of data preparation for AI.
Security Risks and Compliance Issues
Outdated business applications often pose significant security risks and compliance challenges that can derail AI implementation efforts.
Vulnerabilities in Legacy Systems
Legacy systems frequently lack the robust security features found in modern applications, leaving them vulnerable to cyberattacks and data breaches:
- Outdated security protocols: Many older systems use outdated security protocols that are easily compromised.
- Lack of encryption: Sensitive data may not be adequately encrypted, increasing the risk of data breaches.
- Vulnerability to malware: Legacy systems are often more susceptible to malware and other cyber threats.
Investing in robust cybersecurity measures, including regular security audits, penetration testing, and the implementation of up-to-date security protocols, is paramount. Modernizing your security infrastructure is non-negotiable for AI implementation.
Difficulty Meeting Regulatory Compliance
Outdated systems can also make it challenging to meet regulatory compliance requirements related to data privacy and security:
- Difficulties in tracking data usage: Legacy systems may lack the functionality to track data usage, making it difficult to comply with regulations like GDPR and CCPA.
- Inability to meet audit requirements: Auditing data stored in outdated systems can be incredibly complex and time-consuming.
- Lack of data governance features: Many legacy systems lack the features necessary for effective data governance, hindering compliance efforts.
Implementing a comprehensive data governance framework and investing in tools that support compliance with data privacy regulations is essential for responsible and successful AI implementation.
Scalability and Performance Limitations
Legacy systems often lack the scalability and performance capabilities required to support AI applications.
Inefficient Infrastructure and Processing Power
Outdated systems frequently struggle to handle the large datasets and complex computations involved in AI:
- Slow processing speeds: Legacy systems may have slow processing speeds, significantly delaying AI model training and deployment.
- Limited storage capacity: Outdated systems may lack the storage capacity required to store and manage the massive datasets needed for AI.
- Inability to handle large datasets: Legacy systems often struggle to process and analyze the large datasets necessary for effective AI model development.
Migrating to a cloud-based infrastructure provides the scalability and processing power needed to support demanding AI workloads. Cloud computing offers flexibility, elasticity, and the ability to handle significant data volumes.
Lack of Support and Maintenance
Finding support and maintenance for outdated applications can be a significant challenge:
- Difficulty finding skilled technicians: Finding technicians with expertise in legacy systems can be difficult and expensive.
- High maintenance costs: Maintaining outdated systems often involves high maintenance costs, diverting resources from more strategic initiatives.
- Lack of vendor support: Vendors may no longer provide support for outdated applications, leaving organizations vulnerable to security risks and performance issues.
Modernizing your business applications reduces reliance on obsolete technologies, thereby lowering maintenance costs and improving access to skilled support. This ensures the long-term stability and performance of your AI initiatives.
Conclusion
Outdated business apps significantly hamper AI implementation by causing data incompatibility, posing security and compliance risks, and limiting scalability and performance. Clinging to legacy systems can stifle innovation, hinder growth, and compromise your competitive advantage. Don't let outdated business apps stifle your AI journey. Modernize your business apps to unlock the full potential of AI and pave the way for a more efficient, secure, and data-driven future. Assess your current system landscape, identify potential roadblocks, and invest in modernization strategies today. The future of your business depends on it!
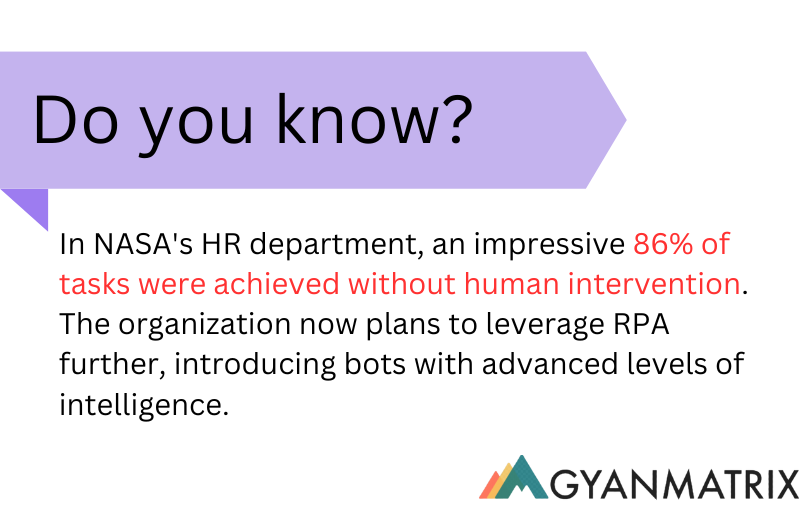
Featured Posts
-
The Channing Tatum Inka Williams Relationship A Comprehensive Look
Apr 30, 2025 -
The Loonies Future Analyzing The Risk Of A Minority Government
Apr 30, 2025 -
Cleveland Guardians Edge Out New York Yankees Bibees Resilience Key
Apr 30, 2025 -
Nevsehir De Olusan Goeruenmez Kaza Yueksekten Duesme
Apr 30, 2025 -
Remy Cointreau Et Son Document Amf Cp 2025 E1029253 Un Apercu
Apr 30, 2025
Latest Posts
-
Pekanbaru Incar Rp 3 6 Triliun Investasi Target Bkpm Tahun Ini
May 01, 2025 -
New Photo Of Prince William A Reflective Moment At Kensington Palace
May 01, 2025 -
Investasi Pekanbaru Bkpm Incar Rp 3 6 Triliun Tahun Ini
May 01, 2025 -
Bkpm Bidik Rp 3 6 Triliun Investasi Di Pekanbaru Tahun 2024
May 01, 2025 -
Aplicativo De Ia Da Meta Uma Alternativa Ao Chat Gpt
May 01, 2025