The Surprising Simplicity Of AI "Thinking": An Analysis Of Current AI Systems
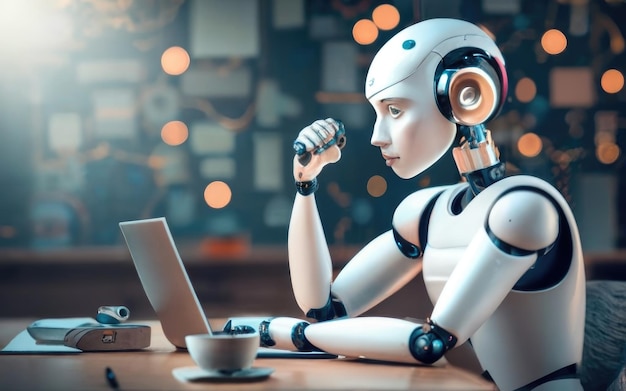
Table of Contents
The Core of AI: Algorithms and Data
At its heart, AI relies on algorithms and data. Algorithms are sets of instructions that tell a computer how to perform a specific task. In the context of AI, these algorithms process vast amounts of data to identify patterns, make predictions, and ultimately, generate outputs. Different types of algorithms underpin various AI applications. Machine learning algorithms allow systems to learn from data without explicit programming, while deep learning models, a subset of machine learning, utilize artificial neural networks with multiple layers to analyze complex data structures.
The importance of high-quality data in AI cannot be overstated. The effectiveness of any AI system hinges on the data it's trained on.
- Data quantity and quality directly impact performance: More data generally leads to better performance, but the quality of that data is paramount. Noisy, incomplete, or inconsistent data will result in inaccurate or unreliable AI outputs.
- Bias in data can lead to biased AI outputs: If the training data reflects existing societal biases, the AI system will likely perpetuate and even amplify those biases. This is a crucial concern in areas like facial recognition and loan applications.
- Data preprocessing is a crucial step: Before an AI system can use data, it needs to be cleaned, transformed, and prepared. This preprocessing step is critical for ensuring the accuracy and reliability of the AI's results. This includes handling missing values, removing outliers, and converting data into a suitable format for the chosen machine learning algorithms.
Keywords: machine learning algorithms, deep learning models, data training, AI datasets, algorithmic bias.
Dissecting "Decision-Making": How AI Arrives at Conclusions
It's crucial to understand that AI doesn't "think" in the human sense. It doesn't possess consciousness or subjective experiences. Instead, AI systems follow pre-defined rules and processes to process information and generate outputs. These processes often involve pattern recognition and statistical analysis. The AI identifies patterns in the data it has been trained on and uses these patterns to make predictions or decisions.
Let's illustrate with examples:
- Image recognition: An AI identifies an object in a picture by comparing its features (edges, shapes, colors) to patterns learned from a vast dataset of labeled images.
- Natural Language Processing (NLP): AI understands and responds to human language by analyzing the grammatical structure, semantics, and context of text or speech. This allows chatbots to converse, machine translation to work, and sentiment analysis to gauge public opinion.
- Recommendation systems: These AI-powered systems suggest products or services by analyzing user preferences, purchase history, and other relevant data. They identify patterns in user behavior to predict what items or services a user might be interested in.
Keywords: AI decision-making processes, pattern recognition algorithms, AI image processing, natural language understanding (NLU), AI recommendation engines.
Limitations of Current AI Systems: Where "Thinking" Falls Short
Despite impressive advancements, current AI systems have significant limitations. They lack general intelligence, the ability to learn and apply knowledge across diverse domains, unlike humans. Common sense reasoning, adaptability to novel situations, and the capacity for creative problem-solving are areas where AI falls considerably short. Explainability and transparency remain major challenges; understanding the reasoning behind an AI's decision can be extremely difficult, leading to concerns about accountability and trust.
Specific limitations include:
- AI struggles with situations outside its training data: An AI trained on images of cats will likely fail to recognize a dog, unless specifically trained to do so.
- AI systems can be easily fooled by adversarial attacks: Subtle changes to an image or input data can cause an AI system to misclassify or make erroneous predictions.
- Understanding the reasoning behind an AI's decision can be difficult: The complexity of some deep learning models makes it hard to interpret their internal workings, making it challenging to debug errors or understand their decision-making processes.
Keywords: AI limitations, general artificial intelligence (AGI), explainable AI (XAI), AI bias, adversarial examples.
The Future of AI Thinking (Optional)
Future developments in AI may address some of these limitations. More advanced learning methods, improved data management techniques, and the development of more adaptable systems are areas of active research. However, ethical considerations and responsible AI development are crucial as AI systems become increasingly sophisticated and integrated into our lives.
Conclusion: Understanding the Simplicity (and Complexity) of AI Thinking
In conclusion, the "thinking" of current AI systems, while often perceived as magical, is ultimately based on sophisticated but relatively straightforward algorithms and vast amounts of data. They lack the general intelligence, common sense, and adaptability of the human mind. Significant limitations remain, emphasizing the need for continued research and development, alongside careful consideration of the ethical implications. Understanding the surprising simplicity of AI thinking, coupled with recognizing its inherent complexities and limitations, is crucial for navigating the rapidly evolving landscape of artificial intelligence. Further your understanding of AI by exploring [link to relevant resource].
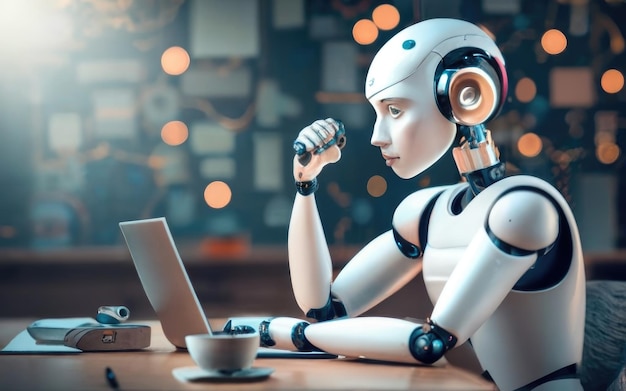
Featured Posts
-
Understanding Willie Nelson Through Fast Facts
Apr 29, 2025 -
Cnn Interview Alan Cumming On His Favorite Childhood Activity In Scotland
Apr 29, 2025 -
Willie Nelsons Annual 4th Of July Picnic Date Location And Tickets
Apr 29, 2025 -
Selling Sunset Star Exposes La Landlord Price Gouging After Fires
Apr 29, 2025 -
The Impact Of Constant Touring On Willie Nelsons Health
Apr 29, 2025
Latest Posts
-
New Music From Willie Nelson Oh What A Beautiful World Album
Apr 29, 2025 -
Oh What A Beautiful World Willie Nelsons Latest Album Details
Apr 29, 2025 -
Willie Nelsons New Album Oh What A Beautiful World
Apr 29, 2025 -
Texas Must See Event Willie Nelsons 4th Of July Picnic
Apr 29, 2025 -
Willie Nelsons Annual 4th Of July Picnic Date Location And Tickets
Apr 29, 2025