Turning "Poop" Into Podcast Gold: An AI-Powered Approach To Repetitive Document Analysis
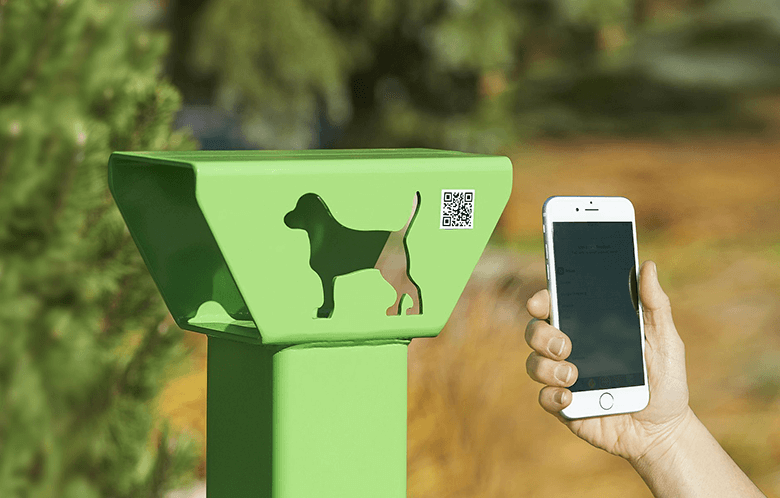
Table of Contents
Identifying the Problem: The Challenges of Manual Repetitive Document Analysis
Manually reviewing large volumes of documents is a significant hurdle in many workflows, especially podcasting. The challenges are multifaceted:
Time Consumption:
Manually reviewing large volumes of documents is incredibly time-consuming, leading to project delays and missed deadlines. Think about:
- Hours spent on manual review: Imagine the countless hours spent manually sifting through transcripts, contracts, and research papers.
- Lost productivity: This time could be spent on more strategic and creative tasks like podcast scripting, guest outreach, or marketing.
- Impact on project timelines: Delays in analysis directly impact the release schedule of your podcast episodes.
Human Error:
Manual analysis significantly increases the risk of human error, leading to inaccurate conclusions and potentially costly mistakes. This includes:
- Overlooking crucial details: Important keywords, dates, or figures can easily be missed during manual review.
- Misinterpretations: Subjectivity in interpretation can skew the results of your analysis.
- Inconsistencies in analysis: Different analysts might draw different conclusions from the same data.
Scalability Issues:
Manual methods struggle to keep up with increasing data volumes. The ever-growing amount of information makes manual processes increasingly inefficient. This manifests as:
- Difficulty handling large datasets: Manual review becomes impractical with large quantities of documents.
- Limitations of manual processes: The sheer volume of data can overwhelm even the most dedicated researcher.
- Need for automation: Automation is essential to handle the growing influx of data efficiently.
AI-Powered Solutions for Efficient Repetitive Document Analysis
Fortunately, AI offers powerful tools to overcome these challenges. Several key technologies are instrumental in automating repetitive document analysis:
Natural Language Processing (NLP):
NLP algorithms are revolutionizing how we process textual data. They can automatically:
- Extract key information: Identify specific data points within documents, such as names, dates, and locations.
- Summarize text: Condense large volumes of text into concise summaries, saving considerable time.
- Identify patterns in large datasets: Uncover trends and insights that would be impossible to detect manually. This includes sentiment analysis, topic modeling, keyword extraction, and entity recognition.
Machine Learning (ML):
ML models, when properly trained, can identify specific patterns and anomalies within documents. This allows for:
- Anomaly detection: Flag unusual or unexpected data points that might require further investigation.
- Predictive modeling: Forecast trends and make data-driven decisions based on past patterns.
- Classification of documents: Automatically categorize documents based on their content, significantly speeding up organization.
Optical Character Recognition (OCR):
OCR technology bridges the gap between physical and digital documents by converting scanned documents and images into searchable text, making them accessible for AI analysis. This results in:
- Digitization of paper documents: Easily incorporate physical documents into your digital workflow.
- Improved data accessibility: Make all your data searchable and readily available for analysis.
- Efficient data processing: Enable AI to process vast amounts of data, regardless of its initial format.
Turning Data into Podcast Gold: Practical Applications
The applications of AI-powered repetitive document analysis extend across various podcasting aspects:
Podcast Research:
Quickly analyze transcripts, identify key themes, and generate talking points for podcast episodes. This includes:
- Efficient topic research: Quickly identify relevant topics and trending subjects.
- Identifying guest experts: Easily find experts by analyzing their published works and interviews.
- Streamlining content creation: Focus on creative aspects, confident that your research is thorough and accurate.
Audience Analysis:
Analyze listener feedback and reviews to understand audience preferences and improve future podcast content. This enables:
- Sentiment analysis of reviews: Gauge audience reaction to your podcasts and identify areas for improvement.
- Identification of popular themes: Discover what resonates with your audience and tailor content accordingly.
- Improvement of podcast strategy: Make informed decisions based on data-driven insights into audience preferences.
Competitor Analysis:
Analyze competitor podcasts to identify trends, gaps in the market, and opportunities for differentiation. This helps in:
- Competitive landscape analysis: Understand the strengths and weaknesses of your competitors.
- Identifying unique selling propositions: Find ways to make your podcast stand out from the crowd.
- Strategic podcast planning: Develop a well-informed strategy based on market analysis.
Choosing the Right AI-Powered Tool for Repetitive Document Analysis
Selecting the appropriate AI tool is crucial for success. Consider these factors:
Consider your budget and specific needs:
- Cost of software: Evaluate different pricing models and choose a solution that fits your budget.
- Integration with existing systems: Ensure seamless integration with your current workflow.
- Scalability options: Choose a tool that can handle your current data volume and future growth.
Look for user-friendly interfaces and strong customer support:
- Ease of use: Select a tool with an intuitive interface that requires minimal training.
- Training resources: Ensure access to comprehensive documentation and tutorials.
- Technical support availability: Choose a vendor that offers reliable and responsive customer support.
Ensure the tool is compatible with your data formats and volume:
- File type compatibility: Verify compatibility with your specific file formats (e.g., PDFs, DOCX, TXT).
- Data processing speed: Ensure the tool can process your data efficiently within a reasonable timeframe.
- Data security features: Prioritize tools with robust security measures to protect sensitive information.
Conclusion:
Transforming the tedious process of repetitive document analysis doesn't have to feel like wading through "poop." By leveraging AI-powered solutions, you can drastically improve efficiency, reduce errors, and unlock valuable insights from your data. This allows you to focus on the creative aspects of podcast production, maximizing your output and transforming your workflow. Start exploring AI-powered tools for repetitive document analysis today and turn your data into podcast gold! Don't let manual analysis hold you back – embrace the power of automation and streamline your repetitive document analysis workflow now.
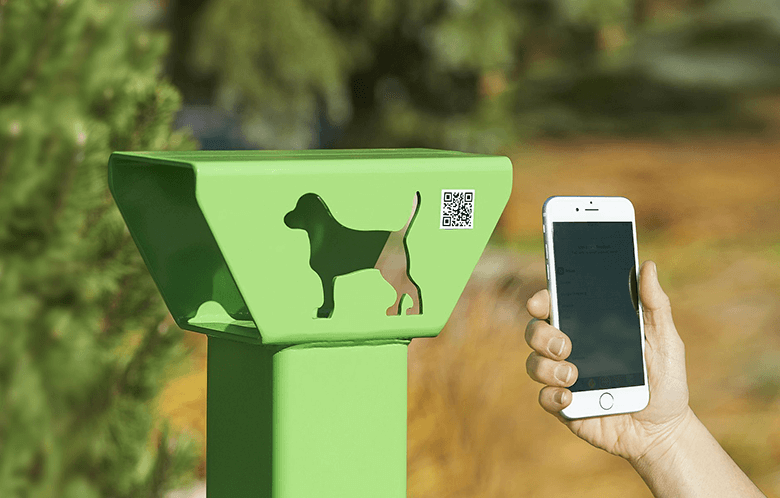
Featured Posts
-
Newsoms Podcast Debut Could Charlie Kirks Interview Be A Political Disaster
Apr 26, 2025 -
Gavin Newsoms Toxic Democrat Remark A Political Backlash
Apr 26, 2025 -
Shedeur Sanders Nfl Future Cam Newtons Prediction And Team Analysis
Apr 26, 2025 -
Pentagon Chaos Exclusive Report On Leaks And Internal Disputes Featuring Pete Hegseth
Apr 26, 2025 -
A Critical Look At Governor Gavin Newsoms Recent Decisions
Apr 26, 2025