Exploring The Cognitive Architecture Of AI: Current Limitations And Future Directions
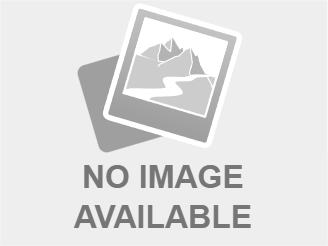
Table of Contents
Current Limitations in AI's Cognitive Architecture
Despite impressive achievements, the cognitive architecture of current AI systems exhibits significant limitations. These shortcomings hinder the development of truly intelligent and adaptable systems.
Lack of Generalization and Transfer Learning
AI systems often excel at specific tasks but struggle to generalize knowledge acquired in one context to another. This contrasts sharply with human intelligence, which displays remarkable flexibility and adaptability.
- Overreliance on large datasets for specific tasks: Current AI models, especially deep learning systems, require massive datasets for training, limiting their ability to learn from smaller, more diverse datasets. This results in specialized, narrow AI rather than general-purpose intelligence.
- Difficulty in applying learned knowledge to novel situations: AI struggles with situations not explicitly covered during training. A system trained to identify cats in one environment might fail to recognize them in a different setting.
- Limited ability to transfer skills between different domains: Knowledge gained in one area (e.g., image recognition) is rarely easily transferable to another (e.g., natural language processing).
- Need for significant retraining when faced with new data or tasks: Adapting an AI system to new tasks often requires extensive retraining, a process that can be time-consuming and resource-intensive. This lack of lifelong learning is a key limitation.
Absence of Common Sense Reasoning and World Knowledge
AI systems lack the extensive, intuitive understanding of the world that humans possess. This "common sense" reasoning is crucial for making realistic inferences and solving complex, real-world problems.
- Difficulty in understanding implicit information and context: Humans effortlessly infer meaning from incomplete information or subtle cues, a capability largely absent in current AI.
- Inability to reason with incomplete or ambiguous data: AI often struggles when presented with uncertain or missing information, unlike humans who can often make reasonable assumptions.
- Limited capacity for causal reasoning and prediction: Understanding cause-and-effect relationships is crucial for problem-solving, but current AI often struggles with causal inference.
- Challenges in integrating diverse sources of information: Humans seamlessly combine information from various sources, while AI systems typically require specialized architectures for each data type.
Limited Explainability and Transparency
Many AI systems, particularly deep learning models, function as "black boxes," making it difficult to understand their decision-making processes. This opacity raises concerns about trust, accountability, and ethical implications.
- Difficulty in identifying biases and errors in AI systems: The lack of transparency makes it challenging to detect and correct biases or errors in AI systems.
- Challenges in debugging and improving AI models: Understanding the reasons behind AI decisions is vital for improving their performance and reliability.
- Concerns about the ethical implications of opaque AI systems: The inability to explain AI decisions raises concerns about fairness, accountability, and potential misuse.
- Need for more explainable AI (XAI) techniques: Research into XAI aims to create more transparent and understandable AI systems.
Future Directions in Cognitive Architecture Research
Overcoming the limitations of current AI requires innovative approaches to cognitive architecture. Several promising directions are emerging:
Neuro-Symbolic AI
This approach aims to integrate symbolic reasoning (manipulating abstract symbols) with connectionist approaches (neural networks) to leverage the strengths of both.
- Development of hybrid models that combine neural networks and symbolic reasoning: These hybrid models aim to combine the strengths of both paradigms, enabling improved reasoning and explainability.
- Incorporation of knowledge representation and reasoning techniques: Symbolic methods allow for explicit knowledge representation and logical reasoning, enhancing AI's understanding and problem-solving abilities.
- Enhanced explainability and transparency through symbolic representations: Symbolic representations can make AI's decision-making processes more transparent and understandable.
- Improved generalization and transfer learning capabilities: The combination of neural and symbolic methods can lead to improved generalization and transfer learning.
Developmental AI
Modeling the cognitive development of AI systems, mimicking human learning processes, offers a powerful path towards more robust intelligence.
- Building AI systems that learn and adapt throughout their lifespan: Developmental AI focuses on creating systems that continuously learn and adapt over time, much like humans.
- Incorporating principles of developmental psychology into AI design: This approach borrows insights from how children learn and develop cognitive abilities.
- Emphasis on interactive learning and exploration: Developmental AI emphasizes the importance of interaction and exploration in the learning process.
- Creation of more robust and adaptable AI systems: Systems developed through this approach are expected to be more resilient and adaptable to changing environments.
Embodied AI
Developing AI systems that interact with the physical world can provide valuable experience and knowledge through direct interaction.
- Building robots with advanced sensory and motor capabilities: Embodied AI requires robots equipped with sophisticated sensors and actuators for interaction with the environment.
- Utilizing reinforcement learning and other methods for physical learning: Learning through interaction relies on reinforcement learning and other techniques to guide the learning process.
- Exploring the role of embodiment in cognitive development: Research investigates how embodiment influences the development of cognitive abilities.
- Development of more robust and adaptable AI systems capable of real-world interaction: Embodied AI aims to create systems capable of seamlessly interacting with and adapting to real-world environments.
Conclusion
The cognitive architecture of AI is a dynamic and complex field. While current AI systems have achieved impressive milestones, significant limitations persist in areas such as generalization, common sense reasoning, and explainability. Future directions like neuro-symbolic AI, developmental AI, and embodied AI offer exciting possibilities for overcoming these obstacles and building more human-like intelligent systems. Continued research and development in the cognitive architecture of AI are crucial to realizing the full potential of this transformative technology and ensuring its responsible and beneficial use. Let's continue to explore and innovate in the field of AI cognitive architecture to build a future powered by truly intelligent systems. Invest in the future of AI cognitive architecture – the possibilities are limitless.
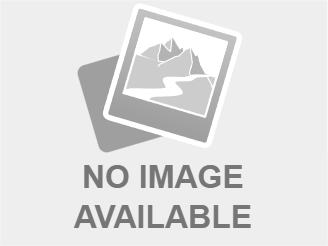
Featured Posts
-
Schumer Stays Put No Passing The Torch Just Yet
Apr 29, 2025 -
Trumps Transgender Athlete Ban Us Attorney General Issues Warning To Minnesota
Apr 29, 2025 -
Alan Cumming Shares Beloved Childhood Memory From Scotland
Apr 29, 2025 -
Why The Venture Capital Secondary Market Is Booming
Apr 29, 2025 -
Wrexhams Promotion Ryan Reynolds Joins The Celebration
Apr 29, 2025
Latest Posts
-
Choosing Between One Plus 13 R And Pixel 9a Performance Camera And Value
Apr 29, 2025 -
One Plus 13 R And Pixel 9a Feature By Feature Review And Recommendation
Apr 29, 2025 -
Is The One Plus 13 R Worth It Comparing It To The Google Pixel 9a
Apr 29, 2025 -
One Plus 13 R Review A Practical Assessment Against The Pixel 9a
Apr 29, 2025 -
One Plus 13 R Vs Pixel 9a A Detailed Comparison Review
Apr 29, 2025