Avoid These App Mistakes To Achieve Your AI Goals
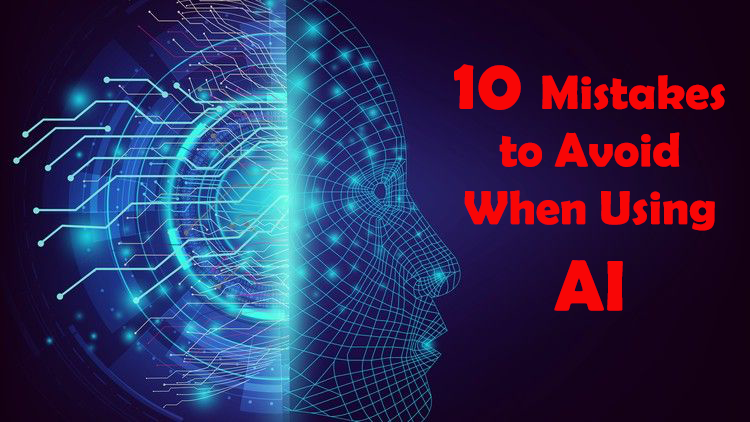
Table of Contents
Failing to Integrate Data Effectively for AI Success
High-quality data is the lifeblood of any successful AI application. Without it, even the most sophisticated algorithms will produce inaccurate and unreliable results. Effective AI app functionality hinges on having clean, relevant, and well-structured data readily accessible. This requires a robust data integration strategy. Failing to establish this foundational element is a significant hurdle to overcome.
- Insufficient data cleaning leads to inaccurate AI predictions. Garbage in, garbage out – this adage rings especially true for AI. Inaccurate, incomplete, or inconsistent data will lead to flawed models and unreliable predictions, undermining the entire purpose of your AI investment.
- Poor data structure hinders efficient AI processing. Data needs to be organized and structured in a way that the AI algorithms can easily process and understand. Inconsistent formats, missing values, and poorly defined data types will significantly slow down processing and reduce accuracy.
- Lack of data variety limits the AI's ability to learn and adapt. A diverse dataset is essential for training robust and generalizable AI models. Limited data variety will result in an AI system that performs poorly when faced with new, unseen data.
Successful data integration strategies leverage techniques like Extract, Transform, Load (ETL) processes to consolidate data from various sources. Cloud-based data warehousing solutions offer scalable and efficient storage and management of large datasets, facilitating seamless integration with your AI applications.
Ignoring the User Experience (UX) in AI App Development
While powerful algorithms are the engine of your AI application, the user experience (UX) is the steering wheel. A poorly designed interface, no matter how sophisticated the underlying AI, will lead to low adoption rates and ultimately, failure. Users need intuitive, clear, and efficient interactions to fully benefit from your AI solution. Neglecting this critical aspect is a common mistake.
- Complex interfaces frustrate users and lead to low adoption rates. Overly complicated interfaces, filled with jargon and confusing navigation, will drive users away. Simplicity and ease of use are paramount.
- Lack of clear explanations of AI functionalities reduces user trust and understanding. Users need to understand how the AI works and what its capabilities and limitations are. Transparency builds trust and encourages adoption.
- Poor feedback mechanisms prevent user engagement and improvements. Gathering user feedback is essential for continuous improvement. Without a mechanism for gathering and acting on feedback, your AI app is unlikely to meet user needs effectively.
Design principles for intuitive AI interfaces include clear visual representations of data, simple navigation, personalized experiences, and helpful tooltips. Prioritizing UX from the initial design stages is crucial for maximizing the value of your AI investment.
Neglecting Security and Privacy in AI App Development
AI applications often handle sensitive data, making security and privacy paramount concerns. Neglecting these critical aspects can lead to devastating consequences, including data breaches, unauthorized access, legal repercussions, and reputational damage. Algorithmic bias is another serious concern; it can lead to unfair or discriminatory outcomes.
- Inadequate data encryption leaves sensitive information vulnerable. Robust encryption is crucial for protecting data both in transit and at rest.
- Lack of user consent management violates privacy regulations. Complying with regulations like GDPR and CCPA is essential for maintaining user trust and avoiding legal penalties.
- Bias in algorithms can lead to unfair or discriminatory outcomes. Careful consideration of potential biases in data and algorithms is essential to ensure fairness and equity.
Best practices for securing AI apps include implementing robust authentication mechanisms, employing strong data encryption, conducting regular security audits, and ensuring compliance with all relevant data privacy regulations. Security should be an integral part of the design and development process, not an afterthought.
Underestimating the Importance of Ongoing Monitoring and Optimization
Developing an AI application is not a one-time event; it's an ongoing process. Continuous monitoring and optimization are crucial for ensuring the accuracy, efficiency, and effectiveness of your AI system over time. Data patterns change, user needs evolve, and algorithms need to adapt.
- Lack of performance monitoring prevents timely detection of issues. Regular monitoring allows you to identify and address problems before they significantly impact performance or accuracy.
- Failure to adapt to evolving data patterns and user needs limits long-term success. Your AI model needs to be retrained and updated regularly to reflect changes in the data and user requirements.
- Inadequate retraining of AI models reduces their accuracy and effectiveness over time. Regular retraining is key to maintaining the performance and accuracy of your AI system.
Methods for monitoring and optimizing AI apps include using analytics dashboards, conducting A/B testing to compare different model versions, and regularly retraining models with updated data. Continuous improvement is essential for maintaining a high-performing AI application.
Choosing the Wrong AI Technologies and Tools
The choice of AI technologies and tools significantly impacts the success of your application. Selecting the wrong tools can lead to performance bottlenecks, scalability issues, and increased development costs.
- Selecting underpowered hardware can lead to performance bottlenecks. Ensure your hardware infrastructure can handle the computational demands of your AI application.
- Choosing the wrong AI framework may limit scalability and flexibility. Select a framework that is well-suited to your specific needs and offers the flexibility to scale as your application grows.
- Ignoring cloud-based solutions can limit cost-effectiveness and scalability. Cloud solutions offer scalability, cost-effectiveness, and easier deployment.
Factors to consider when choosing AI technologies include scalability, cost, ease of use, integration with existing systems, and the availability of skilled developers. Careful consideration of these factors is crucial for building a successful AI application.
Achieving Your AI Goals by Avoiding Common App Mistakes
Successfully deploying an AI application requires careful planning and execution. Avoiding the common mistakes outlined above – poor data integration, neglecting UX, inadequate security, lack of monitoring and optimization, and choosing the wrong technologies – is essential for achieving your AI goals. Each of these areas plays a critical role in ensuring the success and longevity of your AI system. Remember, a well-designed, secure, user-friendly, and continuously optimized AI application unlocks the true potential of AI, providing valuable insights and driving significant business value. To learn more about successful AI app development and optimizing your AI application, explore resources on data integration best practices, UX design for AI, and AI security protocols. Don't let these common mistakes derail your AI journey; learn from them and build an AI application that delivers on its promise.
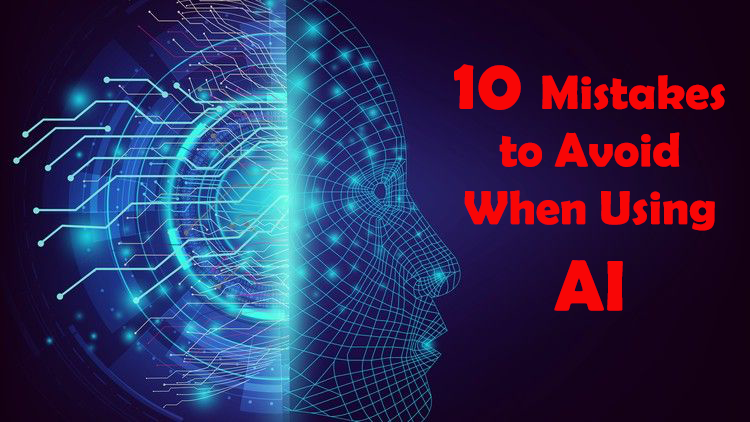
Featured Posts
-
Mercedes Mones Tbs Championship Challenge A Plea To Momo Watanabe
May 01, 2025 -
Xrp Price Prediction Impact Of Sec Lawsuit Outcome On Xrps Value
May 01, 2025 -
App De Ia Da Meta Conheca O Concorrente Do Chat Gpt
May 01, 2025 -
Processo Becciu Ultime Notizie Sui Fondi 8xmille
May 01, 2025 -
Aggiornamenti Sul Caso Becciu L Impatto Delle Preghiere Sulla Decisione Papale
May 01, 2025
Latest Posts
-
Xrp Ripple Investment Strategy Price Below 3
May 02, 2025 -
Xrp Ripple Investment Is It Worth Buying Under 3
May 02, 2025 -
Kshmyrywn Ka Msylh Amn Ke Lye Ansaf Ky Drwrt
May 02, 2025 -
Buy Xrp Ripple Now A Look At The Current Market Under 3
May 02, 2025 -
Should I Invest In Xrp Ripple At Its Current Price Analysis Below 3
May 02, 2025